Agentic AI represents a paradigm shift. It perceives information; understands the context and intent; and autonomously creates, modifies, and orchestrates workflows through contextual reasoning and continual learning. These AI agents enable enterprises to be perpetually adaptable to the dynamic needs of customer and market conditions.
The true revolution emerges in human-AI collaboration and its ability to drive business transformation across industries.
What is Agentic AI?
An Agentic AI framework has the following key components:
- Model - To reason over goals, planning and generating responses
- Tools - To retrieve data and perform actions by invoking an application programming interface (API) or services
- Orchestration - To maintain the memory, state, tools, data acquired/retrieved etc.
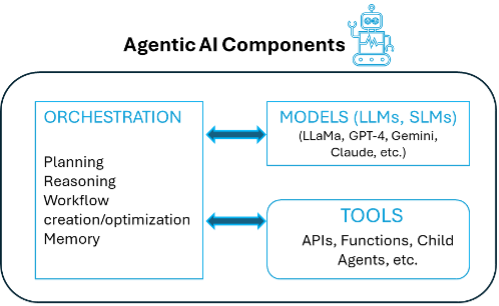
Why the buzz?
Agents have been in existence in various forms such as robotic process automation (RPA), workflows etc., but their applications were limited to non-complex and rule-based tasks that lacked adaptability to dynamic needs and often require human intervention. This is where Agentic AI strikes a perfect symphony for knowledge and complex tasks. With autonomy at its core, Agentic AI moves from an assist role to business transformation.
In today's volatile, competitive and complex business environment, enterprises and businesses are looking to continually adapt. The recent advancements in AI, IoT, robotics, etc. together with the need to drive efficiency and agility, make Agentic AI suitable for various applications across industries. They range from horizontal services such as knowledge management, quality assurance, HR, finance, etc. to industry-vertical services such as underwriting/risk assessment, loan processing, market research, claim processing, fraud detection, clinical management, cyber security, customer servicing, supply chain management, self-driving cars etc.
Agentic AI is enabling a paradigm shift, and new business models are emerging. Enterprises that were focused on the software-as-a-service model are pivoting to service-as-a-software. Also, there is a rise in the number of Agentic AI frameworks such as AutoGen, LangChain, LangGraph, CrewAI, Agentspace etc. to realize this vision.
Four potential applications in industry
Below is a list of a few applications in the healthcare, insurance, manufacturing and technology services industries.
1. Drug discovery – It is a complex problem in the scientific community, which involves years of research, analysis, experimentation and collaboration to arrive at possible solutions such as drug discovery for COVID-19. The challenge is that solutions must adapt to dynamic needs, and new information may become available (such as new variants of COVID-19).
This complex biological problem requires an approach where it can be decomposed into manageable sub-tasks with specialized tools for targeted problem areas (specialized agents, digital twin, research databases, etc.). The process involves brainstorming of ideas (e.g.: brainstorming agent), extracting and synthesizing information from research databases (e.g. search agent), experimental tools such as genome sequencing, analyzing the results (e.g. analysis agent), reasoning the various outcomes simulated using digital twin via techniques such as chain-of-thought, graph-of-thought or tree-of-thought along with feedback loops for continuous learning.
2. Claims Management – It is the core of customer servicing in healthcare and insurance and involves complex process and workflows to determine eligibility, process large datasets such as electronic health records (EHR), X-rays, treatment procedures, diagnosis, recoveries, medical bills, etc. and payouts. For instance, in group benefits (such as disability), this time-consuming problem requires a human-in-the-loop approach to reduce the financial burden and accelerate recovery to participants.
The claim intake agent involves sensors, spatial data about the accident environment, visual language models to analyze the injury details; validation and fraud detection agent to process the claim -- spatial and image analysis, knowledge graph and digital twin to test the hypothesis space. Once a hypothesis is validated, a decision-making agent must weigh in on the job-specific impact, claimant's ability and timelines to recovery and accelerated payout via blockchain. The agent can further actuate its role as a recovery and support agent to continuously monitor the progress, adjust the payout based on progress and optimize recovery to improve overall experience with explainability.
3. Manufacturing - From controlling the flow of production lines to customizing products to making suggestions for improved product design, Agentic AI is likely to have multiple applications in smart manufacturing.
Data from sensors attached to machines, components, and other physical assets in factories and transportation can be analyzed by Agentic AI systems to predict wear-and-tear and production outages, avoiding unscheduled downtime and associated costs to manufacturers. German AI start-up Juna.ai deploys AI agents to run virtual factories, with the aim of maximizing productivity and quality while reducing energy consumption and carbon emissions. It even offers tailored specific goals, such as production agents and quality agents.
4. Technology Services – Enterprises need to be perpetually adaptable, which hinges on speed, quality and cost. Agentic AI will play a prominent role by emulating capabilities of:
- "Requirement analysis agent" such as creating user stories based on standards and template (LLM + RAG)
- "Design agent" to interpret the requirements and create a blueprint based on approved technology, architecture patterns, data flows/source to target mapping (e.g.: for data migration efforts) etc.
- "Data engineering agent" for automated data discovery, build ingestion pipelines leveraging appropriate connectors
- "Data quality agent" for AI/ML driven anomaly detection, de-duplication, self-healing/auto-correction (e.g.: using GIS data wrt location/address anomalies) in conjunction with various tools
- "Synthetic data generator" for test data generation
- Digital twin to create and test hypothesis via "test and learn" simulations, thereby improving productivity and efficiency of data and tech. services/roles.
The way forward
As with any technology advancements, fundamental principles must be applied, such as guardrails for ethics, values, empathy and to address potential bias; explainability and auditability to enable transparency; human-in-the-loop for oversight and decision-making; and accountability on critical areas such as healthcare, financial services etc.,
Human-AI collaboration are to be evaluated closely as this frontier of AI expands its horizons.