How emerging technologies may transform insurance rate modeling
Insurance rate modeling for mass-market consumer products such as P&C, health and life relies heavily on macro risk factors, the “law of large numbers” and building pools of risk. Broadly speaking, outside of specialized lines, relatively little customer-specific data is used in developing rates. Incentives, such as “safe behavior” discounts, are used primarily to encourage good behavior and to help ensure that low-risk prospects do not feel unfairly represented by their premiums. A practical reason for limiting the process to mostly high-level analysis is that large volumes of data are both hard to collect and to analyze on a discrete level. But emerging technologies are starting to remove some of these limitations, potentially creating ways to optimize risk portfolios in consumer-oriented insurance products.
I have written several articles now talking about the potential for the Internet of Things (IoT) in loss prevention and claims facilitation. While much of my focus has been on technologies related to smart homes, arguably more progress has been made in auto telematics and wearables. Data on driving behaviors and personal biometrics of an extraordinary number of people are now being tracked in real time. These data sets may be used to do more than determine the fastest route to work or calculate the remaining target steps you need to take in a day – the data may be a treasure trove of environmental and behavioral information for insurers. Similarly, smart home devices such as connected smoke alarms and leak sensors, along with home security systems, wireless door locks, etc. are beginning to paint a picture of the risk profile in the home at a level never seen before.
But the technology advancements do not stop at the increase in data availability; much of the emerging opportunity has to do with new computing models and “the cloud.” Not long ago, the resources needed to model to an individual rating outweighed the value. But we are now in a world where additional computing resources can be launched with the simple click of a button and disparate databases can easily be joined together for comparison. In other words, the discrete data now exists, and the computing power needed to analyze on an individual level is finally within reach.
See also: How Tech Is Eating the Insurance World
Tiptoeing in
Recognizing that technology may enable improvement on both sides of the risk pool by potentially better identifying both low- and high-risk candidates, insurers are beginning to evaluate options to model risk on a more discrete level. This enhanced lens on data may be one of the most interesting opportunities in the insurance market to-date. The availability of this data, and the associated computing power to process it, is arguably one of the core pillars of the insurtech revolution – but this discussion is for another article. In the meantime, we are seeing early tests toward enhanced data sets in four key markets: health, life, auto and home.
1) Health and Life – Early tests around wearables conducted by major health and life players seemed more to be assessments around consumer comfort with insurers potentially getting a peak into your lifestyle. For example, there have been several examples of fitness trackers given away as affinity products to members of a plan. Initially, there was broad skepticism that consumers would have interest, recognizing that insurers were testing the waters around one-day having access to more detailed lifestyle data. However, early sentiment proved positive, and the market is now seeing the use of individual diagnostic data expanding in the role of premium calculations. Automated collection of this data is not hard to imagine.
2) Auto – Many auto insurers are exploring real-time driving data analysis along with innovative safe driver rates through OBD data collection – with some starting to require it for certain program participation. Consumers, eager to lower their insurance costs, seem to be more than willing to share how fast they drive or how hard they turn when less expensive rates are in play.
3) Home – It’s easy to see how early wins in health, life and auto may translate into the homeowners market. Already, new smart home rates are entering the market, and in these cases smart home products may “self-verify” their presence, removing doubt of whether a customer truly has safety devices installed in the home. As various IoT devices in the home begin to communicate with one another, the insurer has lots of new data that can be used to adjust risk down to a specific premise.
A Virtuous Circle?
In today’s world of rating, there is an imbalance of information that puts insurers at a disadvantage with insureds. Insureds must represent the value of their property, the current state of the property, the cause of loss when it happens, etc. Generally forced to assume that all statements are true, insurers must price uncertainty into the risk. But moving toward greater data transparency may very well be a win-win for both the insurer and the insured. Low-risk customers may be offered rates more in line with their risk profile. High-risk customers may receive higher premiums, but they may also have clear visibility into the factors affecting their rates and potential corrective actions. Insurers may have less volatility in their portfolio with a better understanding of where the losses may occur. Perhaps this increased data availability will result in lower rates for insureds at maintained or even improved margins for insurers.
But how does the overall market respond with more symmetrical information and greater transparency? More importantly, how do consumers respond when they realize the insurer now knows more specific details about them? What if the rating bar moved from basic personal information, like credit score and claims history, to allowing consumers to opt in for very granular inputs such as: how many steps you took today; whether you sped to work; whether you activated your alarm system before leaving your home?
Putting aside the regulatory restrictions, the privacy concerns and the general creepiness of this concept, would consumers be willing to give insurers this very personal data in return for big discounts? If “yes,” would it further ensure good behavior of those that did opt in? Could a “positive self-selection” of sorts start to occur?
In consideration of these potential impacts, there are three economic phenomena that insurers model into rates that may be affected:
1) Adverse selection – People who most need insurance are most likely to buy it, and people less likely to have loss will opt out – e.g., older folks may opt for more health insurance, or safer drivers may choose less coverage than their daredevil counterparts. The bias of high-risk consumers to buy coverage over low-risk consumers results in higher loss ratios and raises premiums of those who participate. But if rates were lowered by removing the risk padding, would lower-risk customers be motivated to participate? Would the risk/reward ratio reach a point where self-insurers feel like the better bet is to participate with the marketplace?
2) Morale hazard – There is risk that insurers bear that insureds, knowing that they have insurance, will be lazy about protecting their belongings. Why lock your doors if insurance would cover a theft? But when behaviors can be monitored, do consumers act differently? Would “safe” people open up data on their personal lives in return for discounts? Perhaps let the insurer know how many nights a week the alarm is armed or the doors are locked for a lowest-rate option?
3) Moral hazard – This phenomena is when insureds take on riskier behavior when coverage is obtained. In other words, a driver who chooses to increase coverage then goes on to take greater driving risks, again, rationalizing the change in behavior as they are “paying for coverage.” Again it’s worth contemplating if behaviors would change by exposing behavioral data.
See also: Embrace Tech Before It Replaces You
Arguably, through increased transparency, a virtuous circle may be created where better information leads to lower rates. Lower rates drive lower-risk candidates into the market; as more lower-risk candidate participate, losses are lessened, which further drives down rates. Additionally, the lowest-risk candidates are the most likely to participate in high-transparency markets, compounding the loss reduction and further driving down rates. Even better, bad actors who know they may not be able to change their behaviors may opt out.
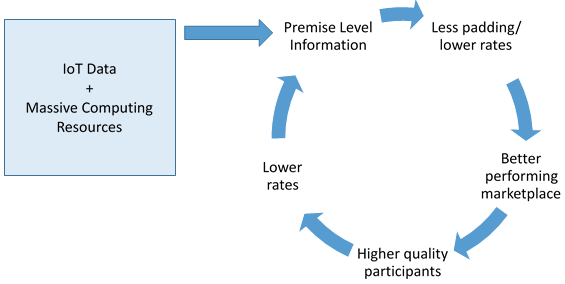
I recognize I am ignoring huge hurdles for this type of transparency: regulatory constraints, privacy issues, consumer interest, etc., but I do feel strongly that early entrants into these types of products may see very interesting results. Basically, better information becomes the great equalizer…
Conclusion
New, high-resolution data sets along with the computing power needed to make them useful are finally here. While having this added information doesn’t necessarily serve as the silver bullet to perfect rate modeling, it certainly offers insurers an opportunity to refine their analysis and reduce the guesswork. Obviously, the effort to operationalize these new data sets may be significant, and, as noted above, there are certainly consumer and regulatory concerns as this highly personal data is used, but the potential is certainly compelling to consider. At the least, now is the time to start considering where these data sets would be useful as the industry contemplates a move toward highly individualized risk opportunities.