For the past 10 years, the insurance industry has been handcuffed by the weather data that’s been available to it – primarily satellite and radar. Although important, these tools leave insurers with a blind spot because they lack visibility into what is happening on the ground. Because of these shortcomings, insurance companies are facing unprecedented litigation and increases in premiums. To solve the problem, we must first review the current situation as well as what solutions have been proposed to resolve this data blind spot.
Why Satellite and Radar Aren’t Enough
While satellites and radar tell us a lot about the weather and are needed to forecast broad patterns, they leave large blind spots when gathering information about exactly what is happening on the ground. Current solutions only estimate what’s happening in the clouds and then predict an expected zone of impact, which can be very different than the actual zone of impact. As many know from experience, it is common for storms to have pockets with more intense storm damage, known as hyper-local storms.
See also: Why Exactly Does Big Data Matter?
The Rise of the Storm-Chasing Contractor
In recent years, the industry has also been beleaguered with a new obstacle: the storm-chasing contractor. These companies target areas that have been hit by a storm with ads on Craigslist and the like. They also exploit insurer’s blind spots by canvassing the area and making homeowners believe there was damage, regardless of whether damage actually occurred. This practice can leave the homeowner with hefty (and unnecessary) bills, hurt the entire industry and lead to higher litigation costs.
Attempts to Solve the Data Blind Spot
Many companies have proposed solutions that aim to solve the insurance industry’s data blind spot. Could a possible solution lie in building better algorithms using existing data? Realistically, if the only improvement made is to the current models or algorithms using existing data, there’s no real improvement because the data the algorithm is using still has gaps. Algorithms will continue to create a flawed output and will have no improved ability to create an actionable result. The answer must lie in a marked improvement in the foundational data.
If better data is required to solve this blind spot, one might think that a crowd-sourced data source would be the best alternative. On the surface, this solution may appear to be a good option because it collects millions of measurements that are otherwise unavailable. The reality is that big data is only relevant when you can build true value out of the entire data set and, while cell phones provide millions of measurements, the resulting cleaned data remains too inaccurate for crowd-sourced weather data to provide a reliable dataset.
The alternative crowd-sourced weather networks that use consumer weather stations to collect data also lead to huge problems in data quality. These weather stations lack any sort of placement control. They can be installed next to a tree, by air conditioning units or on the side of a house – all of which cause inaccurate readings that lead to more flawed output. And although these types of weather stations are able to collect data on rain and wind, none are able to collect data on hail – which causes millions of dollars in insurance claims each year.
The Case for an Empirical Weather Network
To resolve the insurance industry’s blind spot, the solution must contain highly accurate weather data that can be translated into actionable items. IoT has changed what is possible, and, with today’s technology, insurers should be able to know exactly where severe weather has occurred and the severity of damage at any given location. The answer lies in establishing a more cost-effective weather station, one that is controlled and not crowd-sourced. By establishing an extensive network of weather stations with controlled environments, the data accuracy can be improved tremendously. With improved data accuracy, algorithms can be reviewed and enhanced so insurers can garner actionable data to improve their storm response and recovery strategies.
Creating an extensive network of controlled weather stations is a major step toward fixing the insurance industry’s data blind spot, but there is one additional piece of data that is required. It is imperative that these weather stations measure everything, including one of the most problematic and costly weather events – hail. Without gathering hail data, the data gathered by the controlled weather stations would still be incomplete. No algorithm can make up for missing this entire category of data.
See also: 4 Benefits From Data Centralization
While technology has improved tremendously over the past 10 years, many insurers continue to use traditional data that has always been available them. Now is the time for insurers to embrace a new standard for weather data to gain insights that eliminate their blind spot, improve their business and provide better customer experiences.
Understory has deployed micro-networks of weather stations that produce the deep insights and accuracy that insurers need to be competitive today. Understory’s data tracks everything from rain to wind to temperature and even hail. Our weather stations go well beyond tracking the size of the hail; they also factor in the hail momentum, impact angle and size distribution over a roof. This data powers actionable insights
Industry’s Biggest Data Blind Spot
Traditional weather data -- primarily radar and satellite -- leave insurers with a blind spot about what's actually happening on the ground.
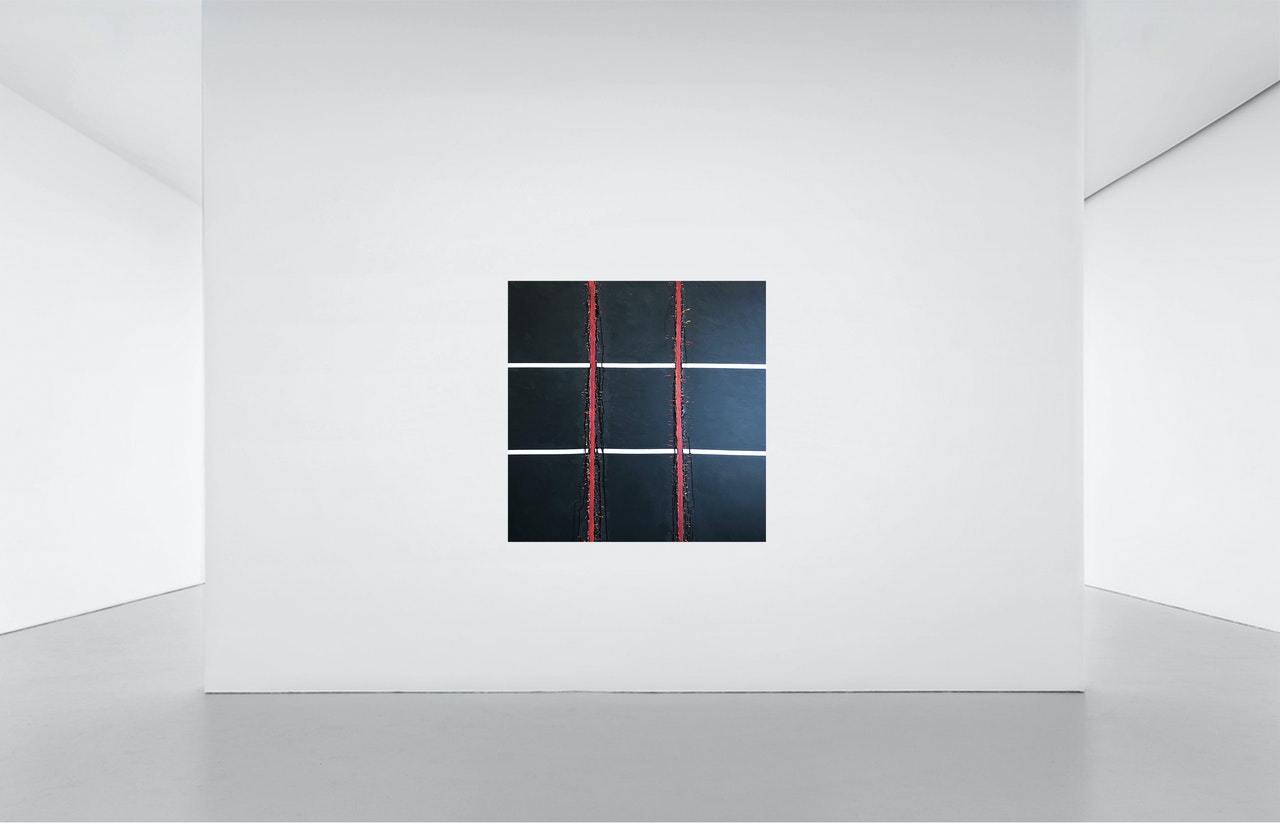